Navigation Menu
Beginners Guide to AI
AI Prompt Engineering
AUTONOVA AI
beta
AI Research Papers
AI|DOCS
Navigation Menu
Beginners Guide to AI
AI Prompt Engineering
AUTONOVA AI
beta
AI Research Papers
AI|DOCS
Navigation Menu
Beginners Guide to AI
AI Prompt Engineering
AUTONOVA AI
beta
AI Research Papers
AI|DOCS
Navigation Menu
Beginners Guide to AI
AI Prompt Engineering
AUTONOVA AI
beta
AI Research Papers
AI|DOCS
Navigation Menu
Beginners Guide to AI
AI Prompt Engineering
AUTONOVA AI
beta
AI Research Papers
AI|DOCS
Navigation Menu
Beginners Guide to AI
AI Prompt Engineering
AUTONOVA AI
beta
AI Research Papers
AI|DOCS
Large Language Models [LLM]
Llama 3.1
Llama 3.1
Meta's groundbreaking release of Llama 3.1, a collection of powerful open-source AI models. These models represent a significant leap in accesible AI technologies. The launch is comprised of a new flaghship 405B parameter model in addition to refreshes to the previously released 8B and 70B models.
Model Sizes + Architecture:
New Flagship Model:
Llama 3.1 - 405B parameter
- Refresh of existing 8B and 70B parameter models
Architecture:
Auto-regressive language model utilising optimised transformer architecture. Incorporates Grouped-Query Attention (GQA) for improved inference scalability
Context Length:
128,000 tokens for all model sizes (16x increase)
Training Data and Approach:
Pretrained Data-Set:
15 trillion tokens (from publicly available sources)
- Fine-tuning data over 25M synthetically generated examples
- Fine-tuning methodology (SFT) + (RLHF)
Data Cut-off:
December 2023
Multilingual Support:
Supported Languages:
English, German, French, Italian, Portuguese, Spanish, Hindi and Thai
- Optimised for dialogue based use-cases.
Performance
How Does Llama 3.1 Stack Up?
Level 3.1 Performance:
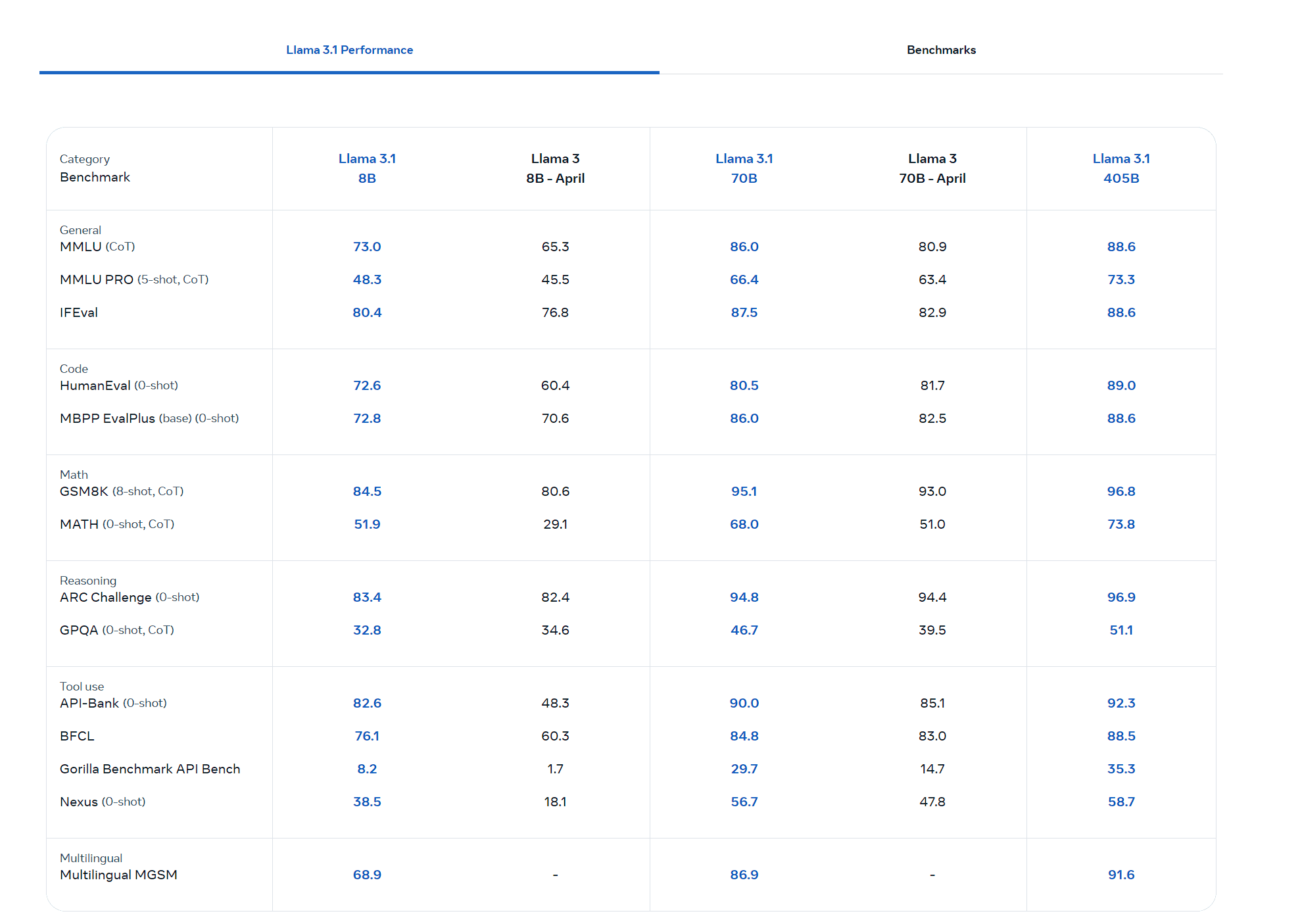
Explanatory Articles:
Fine-Tuning (SFT vs RLHF)
https://medium.com/@veer15/fine-tuning-vs-human-guidance-sft-and-rlhf-in-language-model-tuning-6ad54e1ba628
Model Sizes + Architecture:
New Flagship Model:
Llama 3.1 - 405B parameter
- Refresh of existing 8B and 70B parameter models
Architecture:
Auto-regressive language model utilising optimised transformer architecture. Incorporates Grouped-Query Attention (GQA) for improved inference scalability
Context Length:
128,000 tokens for all model sizes (16x increase)
Training Data and Approach:
Pretrained Data-Set:
15 trillion tokens (from publicly available sources)
- Fine-tuning data over 25M synthetically generated examples
- Fine-tuning methodology (SFT) + (RLHF)
Data Cut-off:
December 2023
Multilingual Support:
Supported Languages:
English, German, French, Italian, Portuguese, Spanish, Hindi and Thai
- Optimised for dialogue based use-cases.
Performance
How Does Llama 3.1 Stack Up?
Level 3.1 Performance:
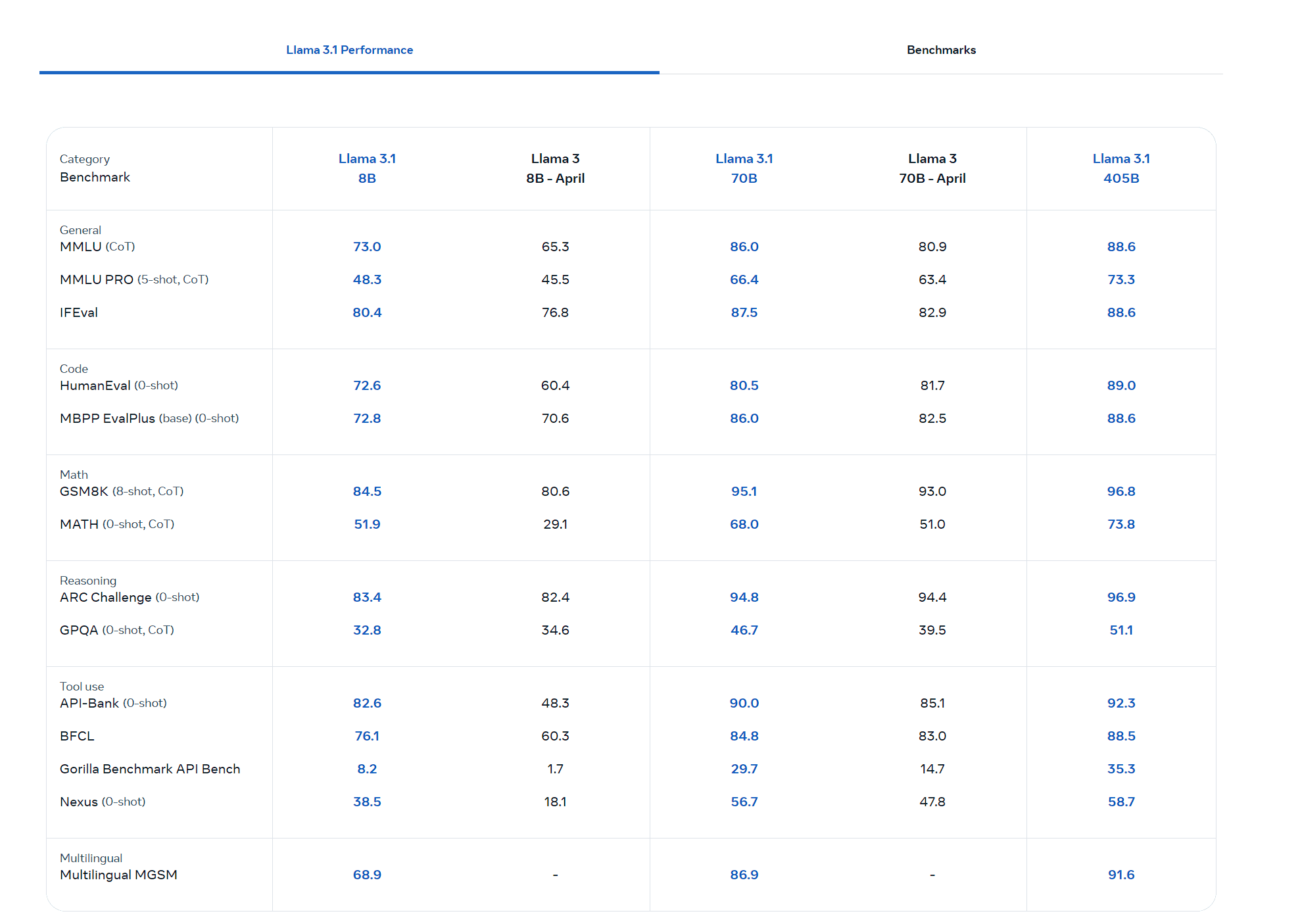
Explanatory Articles:
Fine-Tuning (SFT vs RLHF)
https://medium.com/@veer15/fine-tuning-vs-human-guidance-sft-and-rlhf-in-language-model-tuning-6ad54e1ba628
Model Sizes + Architecture:
New Flagship Model:
Llama 3.1 - 405B parameter
- Refresh of existing 8B and 70B parameter models
Architecture:
Auto-regressive language model utilising optimised transformer architecture. Incorporates Grouped-Query Attention (GQA) for improved inference scalability
Context Length:
128,000 tokens for all model sizes (16x increase)
Training Data and Approach:
Pretrained Data-Set:
15 trillion tokens (from publicly available sources)
- Fine-tuning data over 25M synthetically generated examples
- Fine-tuning methodology (SFT) + (RLHF)
Data Cut-off:
December 2023
Multilingual Support:
Supported Languages:
English, German, French, Italian, Portuguese, Spanish, Hindi and Thai
- Optimised for dialogue based use-cases.
Performance
How Does Llama 3.1 Stack Up?
Level 3.1 Performance:
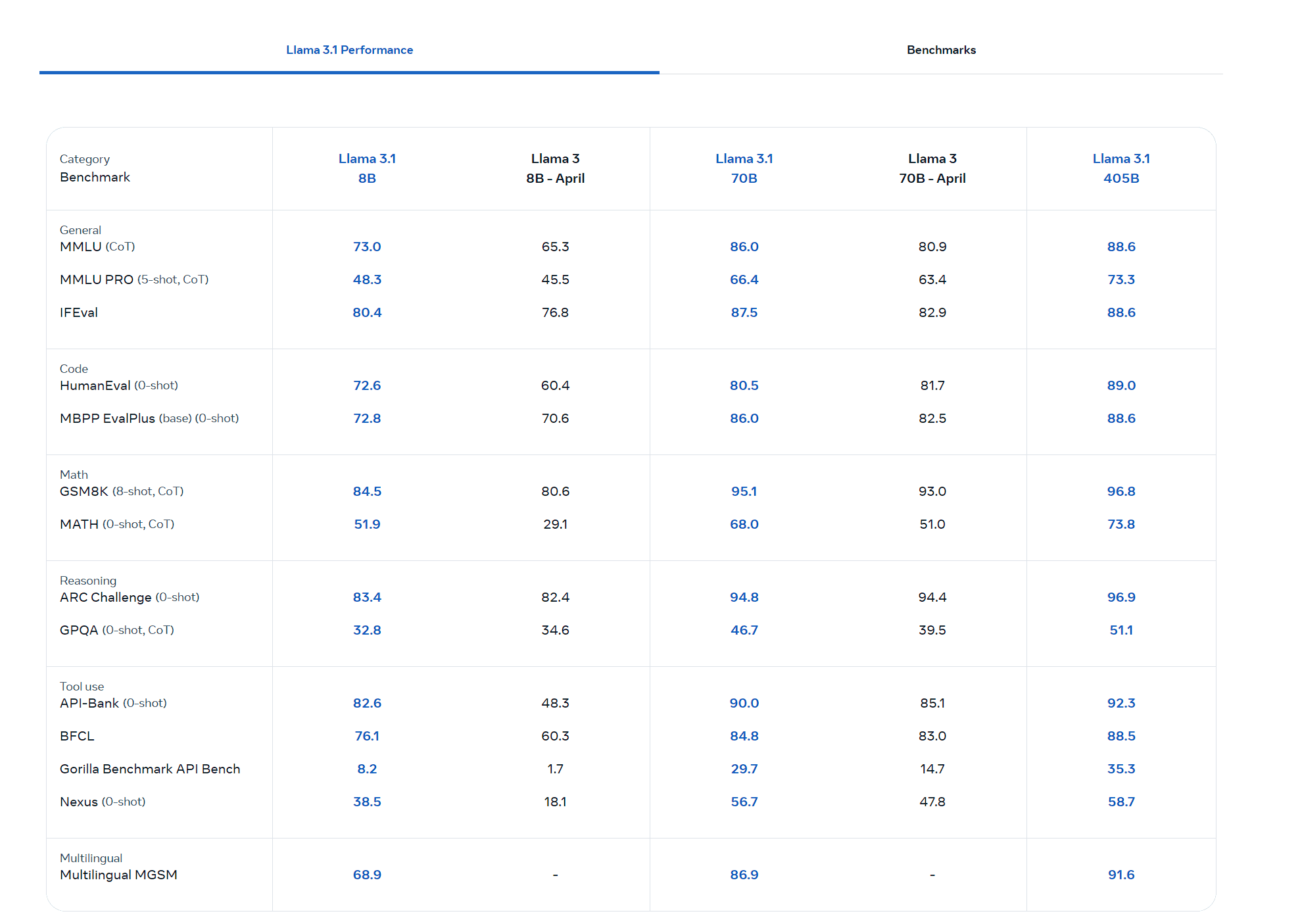
Explanatory Articles:
Fine-Tuning (SFT vs RLHF)
https://medium.com/@veer15/fine-tuning-vs-human-guidance-sft-and-rlhf-in-language-model-tuning-6ad54e1ba628
Model Sizes + Architecture:
New Flagship Model:
Llama 3.1 - 405B parameter
- Refresh of existing 8B and 70B parameter models
Architecture:
Auto-regressive language model utilising optimised transformer architecture. Incorporates Grouped-Query Attention (GQA) for improved inference scalability
Context Length:
128,000 tokens for all model sizes (16x increase)
Training Data and Approach:
Pretrained Data-Set:
15 trillion tokens (from publicly available sources)
- Fine-tuning data over 25M synthetically generated examples
- Fine-tuning methodology (SFT) + (RLHF)
Data Cut-off:
December 2023
Multilingual Support:
Supported Languages:
English, German, French, Italian, Portuguese, Spanish, Hindi and Thai
- Optimised for dialogue based use-cases.
Performance
How Does Llama 3.1 Stack Up?
Level 3.1 Performance:
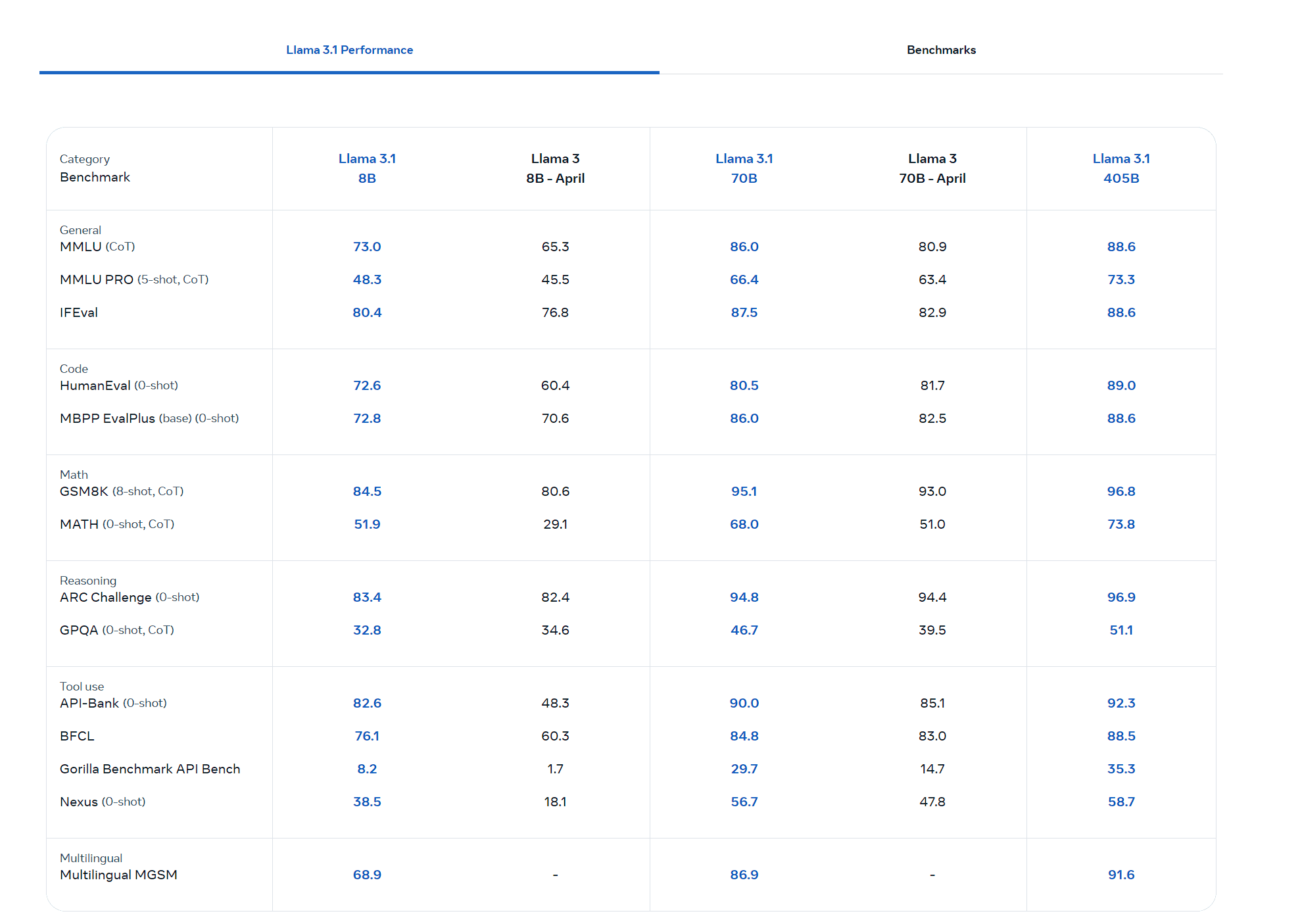
Explanatory Articles:
Fine-Tuning (SFT vs RLHF)
https://medium.com/@veer15/fine-tuning-vs-human-guidance-sft-and-rlhf-in-language-model-tuning-6ad54e1ba628
Model Sizes + Architecture:
New Flagship Model:
Llama 3.1 - 405B parameter
- Refresh of existing 8B and 70B parameter models
Architecture:
Auto-regressive language model utilising optimised transformer architecture. Incorporates Grouped-Query Attention (GQA) for improved inference scalability
Context Length:
128,000 tokens for all model sizes (16x increase)
Training Data and Approach:
Pretrained Data-Set:
15 trillion tokens (from publicly available sources)
- Fine-tuning data over 25M synthetically generated examples
- Fine-tuning methodology (SFT) + (RLHF)
Data Cut-off:
December 2023
Multilingual Support:
Supported Languages:
English, German, French, Italian, Portuguese, Spanish, Hindi and Thai
- Optimised for dialogue based use-cases.
Performance
How Does Llama 3.1 Stack Up?
Level 3.1 Performance:
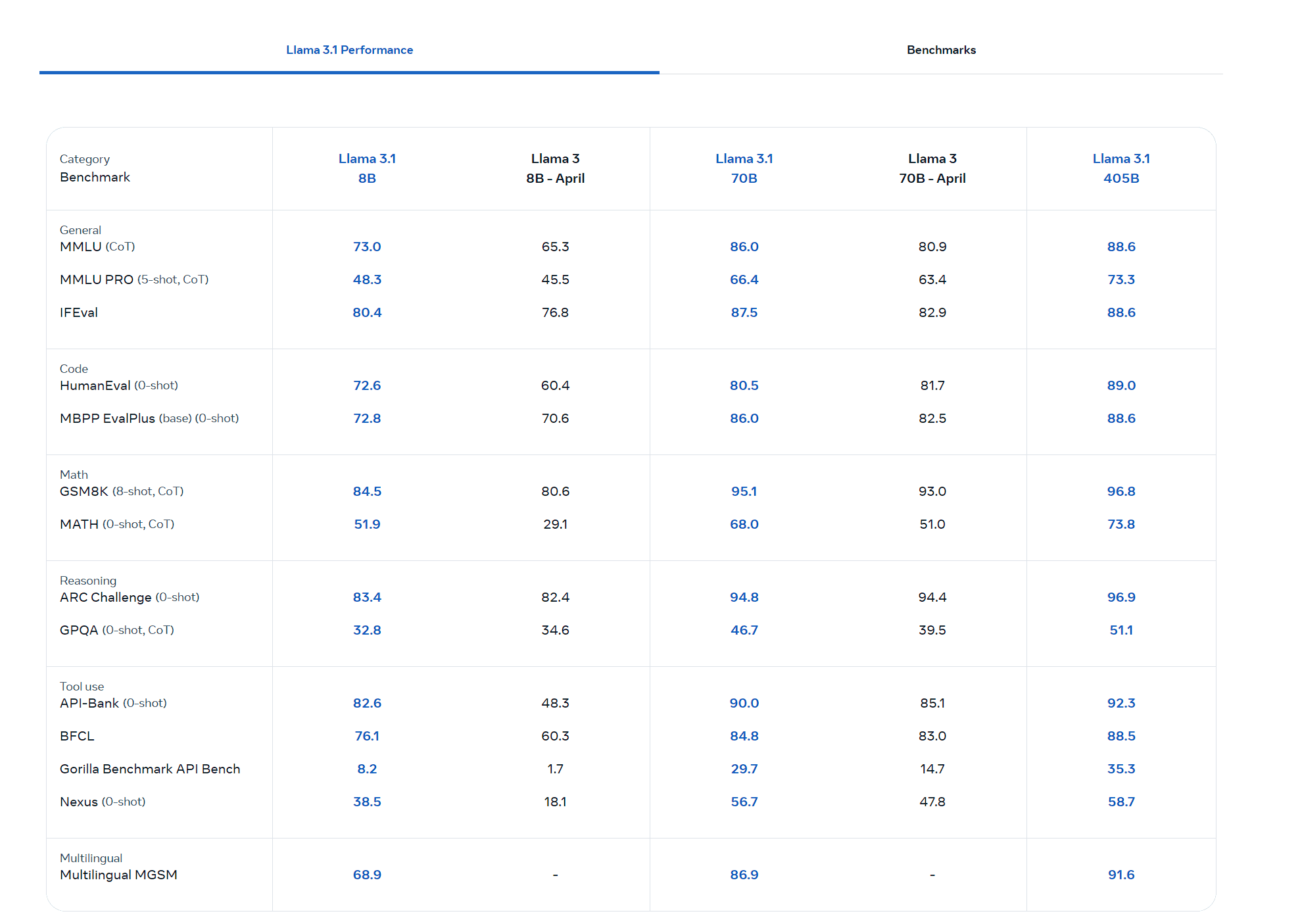
Explanatory Articles:
Fine-Tuning (SFT vs RLHF)
https://medium.com/@veer15/fine-tuning-vs-human-guidance-sft-and-rlhf-in-language-model-tuning-6ad54e1ba628
Copy To Clipboard ->
Lummi AI
© Copyright 2024. All rights reserved.